Selecting studies and data extraction
Ensuring the right articles are included in your evidence review
Selecting studies is a multi-step process requiring methodical, sometimes subjective decisions and meticulous documentation. A careful approach is critical to ensure that bias is minimised and that the reader can trace the methods used to decide whether a study meets the inclusion criteria of the review or guideline.
This is probably the most time-consuming phase of the evidence review as you may be starting with thousands of records to process. Much effort has been made to create tools that partly automate this step. These automation tools are continually being refined but rely on a well-constructed PI/ECO framework to assist with algorithmic screening of titles and abstracts (Tsafnat, 2014; Marshall, 2019; Thomas, 2021). Despite this automation, there are subjective judgements involved and it is important that the reviewer has some knowledge of the topic area, or can consult with a content expert, and that the final selection of studies for the review is undertaken by more than one author.
Chapter 4 of the Cochrane Handbook provides more detail on the process of selecting studies for systematic reviews including advice on how to prepare for this process. Assessing the risk of bias is a fundamental step once studies have been selected for inclusion in the review, and is discussed separately in the Assessing the risk of bias module.
To standardise the study selection process, it is recommended that the PRISMA (Preferred Reporting Items for Systematic Reviews and Meta-Analyses) flow diagram is used.
What to do
1. Manage the references
As discussed in the Identifying the evidence module, once you have finished your search process and documented your ‘close date’, you will need to prepare for the selection of studies by merging your lists of retrieved records.
Because records will have been retrieved from multiple databases and various other sources there are often duplicates in the merged list. Duplicate records should be removed before proceeding further and this process can be largely automated by using reference management software, such as EndNote, or systematic review management tools, such as Covidence. You may also have to manually sort and remove some duplicates that have been inconsistently entered across different databases — possibly due to different formatting of author names or abbreviations — as these might not be fully picked up in an automated process (see the Identifying the evidence module for more detail on this process). It is important to document the number of duplicates removed as the first step in the PRISMA flow diagram.
Cochrane notes that the study is the unit of interest in a systematic review, not the report. The evidence search may have identified more than one report for a particular study (e.g. protocol, interim analyses, final report, economic analysis, etc.). There is also the possibility of multiple publications of the same study report appearing in different journals, which can be particularly difficult to detect. To systematically organise your evidence base Cochrane recommends linking together multiple reports of the same study, recognising that it may be necessary to correspond with the authors of the reports to clarify any uncertainties (Section 4-6-2 Cochrane Handbook). Once included, information or data used in the review may come from one or more reports of the same study.
2. Establish how you will do the screening
Whether you are commissioning a systematic review or doing it yourself, you should arrange to have at least two independent investigators available to select the final studies for inclusion.
As this is quite a resource-intensive step you may choose to have one person undertake the initial screening (titles and abstracts) and then involve a second person in the full-text screening in order to minimise resource use. For example, this might be the case if the evidence search generates several thousand records to screen.
If single screening of titles and abstracts is the preferred approach, it is recommended that a second person checks a random sample of screened records to increase the reliability of the process. Screening may involve several reviewers. Irrespective of how many people are involved in the initial screening, it is important that they are all very clear on what the criteria are for determining relevance – pilot testing this process on a sample of records at the outset is highly recommended.
The final selection of studies should always be undertaken by more than one person, with the potential involvement of a third person to resolve any disputes about the eligibility of studies.
PRISMA 2020 describes several approaches to selecting studies and discusses the pros and cons of each (see Item 8 of the explanation and elaboration paper). Increasingly, a mix of screening approaches might be applied, such as single or double screening, automation to eliminate records before screening or prioritise records during screening.
3. Screen the titles and abstracts
Using your PICO (or PICO alternative) criteria you need to screen the records for their relevance to the research questions. This involves screening for key information within the title/abstract. Any irrelevant reports or studies retrieved by the evidence search can quickly be removed, however any studies where you are unsure whether it is relevant or not should be retrieved for further examination.
Make sure the PRISMA checklist is used with details displayed in a PRISMA flow diagram. There are different PRISMA flow diagrams to choose from depending on whether the systematic review is a new or updated review, and whether the search includes other sources in addition to databases and registers. Figure 1 shows the PRISMA flow diagram for updated systematic reviews that include searches of databases, registers and other sources.
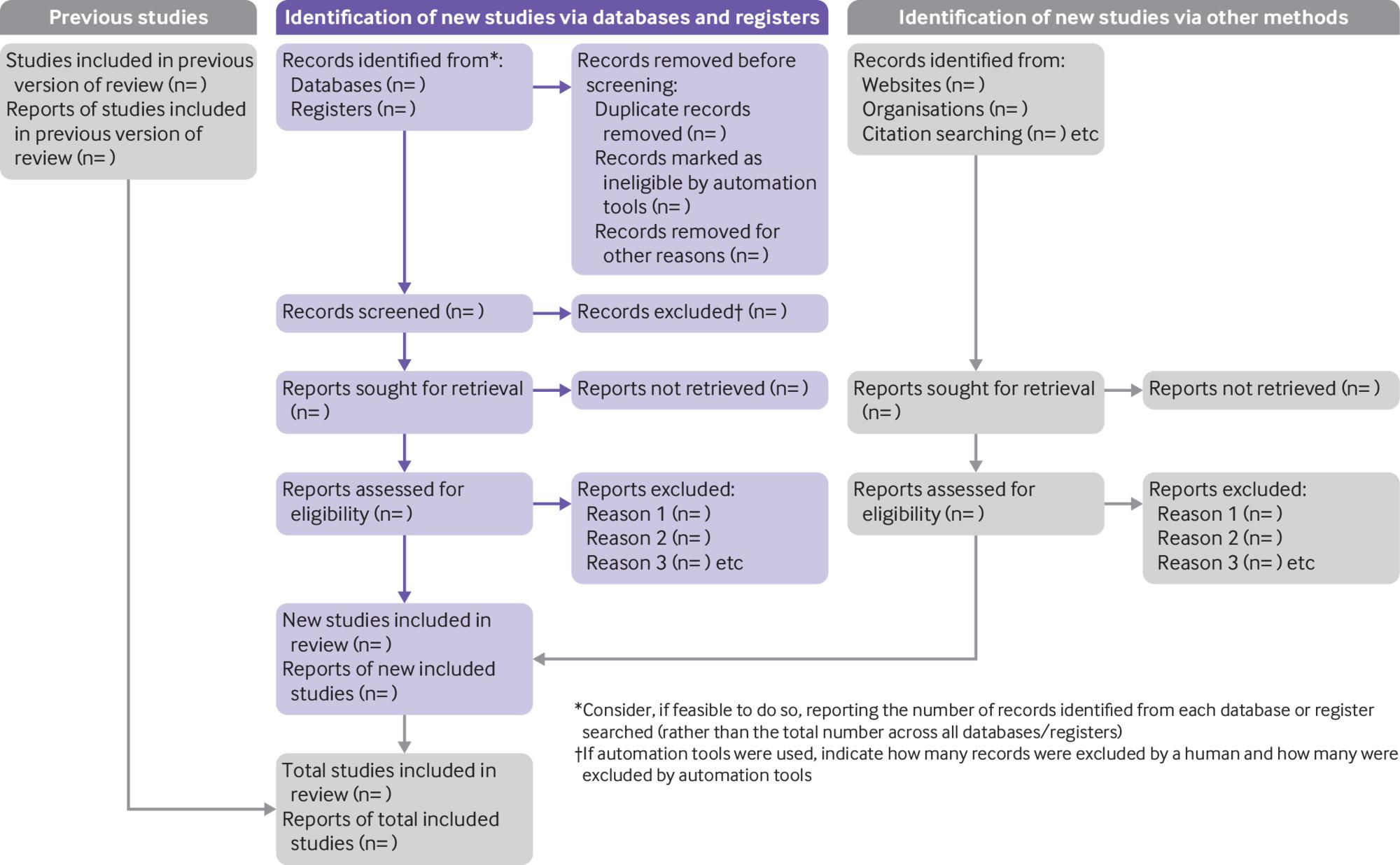
From: Page MJ, McKenzie JE, Bossuyt PM, Boutron I, Hoffmann TC, Mulrow CD, et al. The PRISMA 2020 statement: an updated guideline for reporting systematic reviews. BMJ 2021;372:n71. doi: 10.1136/bmj.n71. For more information, visit: http://www.prisma-statement.org/
Automation tools have been developed to help with screening decisions. Abstrackr and Rayyan are now free to use (with logins) but there are also commonly used commercial tools such as Covidence, EPPI-Reviewer, and Distiller SR. These tools have various functions including inclusion and exclusion criteria management, priority screening (whereby unscreened records are continually assessed for relevance), deployment of machine learning classifiers, and can display disagreements and generate PRISMA flow diagrams (Tsafnat, Glasziou et al. 2014; Marshall, 2019; Gates, 2019).
4. Retrieve the full-text articles and assess eligibility
At this stage you will have a set of reports that you have decided have some relevance to the question and the selection criteria. You will need to retrieve the full-text versions of these reports to examine their content. Tracking down the full text can be time-consuming but tools such as EndNote and Covidence can help with this task, either by automatically capturing the full text or by linking to the article’s unique digital object identifier (DOI).
You need to carefully apply the inclusion and exclusion criteria against the full text of each report, typically focusing on the methods section, and document whether it meets the criteria and if not why it does not meet the criteria. This is the key step where judgements are made about whether the study can be included in your evidence synthesis. This is also the point in the process when multiple reports to the same study can be linked together.
It is essential that at least two people conduct this process independently and reach consensus on the decisions. At least one reviewer needs to be familiar with the content to be able to make these judgements. Any disagreement between investigators can be resolved through discussion or by obtaining a third opinion and should be documented. Several tools, such as those already mentioned, can facilitate this process by easily identifying discordant judgments and recording the reason for exclusion when required.
You will need to record the specific reason for excluding studies that a reader might plausibly expect to see among the included studies in a table of excluded studies and document it in the PRISMA flow diagram (section 4-6-5 of the Cochrane Handbook). This table is likely to be reviewed during public consultation when people question why some studies were not included in the final guideline.
If studies published in languages other than English are to be included, services like Google Translate can help determine eligibility (and reasons for exclusion) and help decide whether additional translation is warranted. Non-English studies that meet the inclusion criteria for which it is not possible to extract the relevant information or data should be classified as ‘studies awaiting assessment’ rather than ‘excluded studies’ (section 4-4-5 of the Cochrane Handbook).
5. Pilot your data extraction form
Data extraction is the process of sourcing and recording relevant information from the final set of eligible studies. You need to use a form that standardises the extraction of data that suits the particular circumstances of your review. Reviewers should agree on the form that will be used prior to the data extraction process and pilot it against three to four studies to ensure it is fit for purpose. This can highlight any potential for confusion in coding instructions and helps shape a standardised extraction tool. If you can, use two people to extract the data independently but it is probably more feasible to have one person extract the data and another person to review it.
Information you might extract includes: title, authors, reference/source, country, year of publication, setting, sample size, study design, participants (age, sex, inclusion/exclusion criteria, withdrawals/losses to follow-up, subgroups), intervention details, outcome measures, duration of follow-up, point estimates and results of clinically relevant outcomes.
A detailed checklist of items to consider in data collection is available in section 5-3 of the Cochrane Handbook.
Although many reviewers typically use tables or spreadsheets to collect data, several of the systematic review management tools referred to earlier have data extraction capability. Setting up data extraction forms in these systems will require some upfront investment of time but is worth considering for large reviews. The Systematic Review Toolbox provides a comprehensive catalogue of tools that support data extraction.
6. Extract data from the selected studies
The final set of studies will be those that you and your co-reviewers decided met the criteria for inclusion. Extracting relevant data from these studies is the next step in the process and depends on what evidence is relevant to your question. Outcome data should be extracted as originally presented in the studies, being careful to note the sample size for each timepoint data are collected for, as this is likely to change as the duration of follow up increases. Not all outcomes and results will be measured and reported in the same way across studies so it is important that you are clear about what information you are collecting (e.g. unit of measurement, scale length and direction, measure of variance reported). This will help in making best use of the data you have and in preparing your data for synthesis (See the Synthesising the evidence module).
The focus during data extraction will be on outcomes relevant to the review question, however you need to be open to unexpected findings, particularly around the reporting of harms or adverse effects. Likewise, you should be careful not to automatically exclude studies on the basis that outcomes of interest are not reported as these outcomes may have been measured but not reported. This is referred to as selective non-reporting of results or ‘outcome reporting bias’ (see section 13-2 of the Cochrane Handbook for approaches for dealing with this issue).
It is important that the study design of each article is documented so that an appropriate tool can be used to assess the risk of bias. Most studies declare the study design in the title or ‘methods’ section of an article, however this should be confirmed on examination of the study given the complexity of some study designs, particularly non-randomised studies. There are several tools available for classifying study designs which are usually framed around guiding questions to help investigators decide how to categorise types of study design, for example, the AHRQ Tool for the Classification of Study Designs, or the NICE Algorithm for classifying quantitative study designs.
Finally, it may be necessary to contact study investigators to obtain unreported or missing data, or to clarify other aspects of the conduct of the study. If you do contact investigators, be clear about the data or information you need, ask for descriptions rather than yes/no answers (especially in relation to methods) and consider providing a table for investigators to complete.
Useful resources
Methods
PRISMA (Preferred Reporting Items for Systematic Reviews and Meta-Analyses) Checklist
Cochrane Handbook Chapter 4: Searching and selecting studies
Cochrane Handbook Chapter 5: Collecting data
Agency for Healthcare Research and Quality (AHRQ) Tool for the Classification of Study Designs
Centre for Reviews and Dissemination’s (CRD’s) guidance for undertaking reviews in health care
Systematic Review Toolbox - use the Study Selection and Data Extraction checkboxes to find more tools for selecting studies and data extraction.
Tools
References
Gates A, Guitard S, Pillay J, Elliott SA, Dyson MP, Newton AS, Hartling L. Performance and usability of machine learning for screening in systematic reviews: a comparative evaluation of three tools. Syst Rev. 2019 Nov 15;8(1):278. Doi: 10.1186/s13643-019-1222-2. PMID: 31727150; PMCID: PMC6857345.
Higgins JPT, Thomas J, Chandler J, Cumpston M, Li T, Page MJ, Welch VA (editors). Cochrane Handbook for Systematic Reviews of Interventions version 6.2 (updated February 2021). Cochrane, 2021. Available from www.training.cochrane.org/handbook.
Lefebvre C, Glanville J, Briscoe S, Littlewood A, Marshall C, Metzendorf M-I, Noel-Storr A, Rader T, Shokraneh F, Thomas J, Wieland LS. Chapter 4: Searching for and selecting studies. In: Higgins JPT, Thomas J, Chandler J, Cumpston M, Li T, Page MJ, Welch VA (editors). Cochrane Handbook for Systematic Reviews of Interventions version 6.0 (updated July 2019). Cochrane, 2019. Available from www.training.cochrane.org/handbook.
Marshall, I. J. and B. C. Wallace (2019). "Toward systematic review automation: a practical guide to using machine learning tools in research synthesis." Systematic Reviews 8(1): 163.
Page MJ, McKenzie JE, Bossuyt PM, Boutron I, Hoffmann TC, Mulrow CD, et al. The PRISMA 2020 statement: an updated guideline for reporting systematic reviews. BMJ 2021;372:n71. doi: 10.1136/bmj.n71. For more information, visit: http://www.prisma-statement.org/
Reid C. Robson, Ba’ Pham, Jeremiah Hwee, Sonia M. Thomas, Patricia Rios, Matthew J. Page, Andrea C. Tricco (2019). "Few studies exist examining methods for selecting studies, abstracting data, and appraising quality in a systematic review." J Clin Epidemiol 106: 121-135 https://doi.org/10.1016/j.jclinepi.2018.10.003.
Seo, H. J., S. Y. Kim, et al. (2016). "A newly developed tool for classifying study designs in systematic reviews of interventions and exposures showed substantial reliability and validity." J Clin Epidemiol 70: 200-205.
Thomas J, McDonald S, Noel-Storr A, Shemilt I, Elliott J, Mavergames C, Marshall IJ. Machine learning reduced workload with minimal risk of missing studies: development and evaluation of a randomized controlled trial classifier for Cochrane Reviews. J Clin Epidemiol. 2020 Nov 7:S0895-4356(20)31172-0. doi: 10.1016/j.jclinepi.2020.11.003. Epub ahead of print. PMID: 33171275.
Tsafnat, G., P. Glasziou, et al. (2014). "Systematic review automation technologies." Systematic reviews 3(1): 74.
Acknowledgements
NHMRC would like to acknowledge and thank Steve McDonald from Cochrane Australia and Miyoung Choi for their contributions to this module.
Public consultation Version 3.3 was last updated on 27 September 2021.
Suggested citation: NHMRC. Guidelines for Guidelines: Selecting studies and data extraction. https://www.nhmrc.gov.au/guidelinesforguidelines/develop/selecting-studies-and-data-extraction. Last published 27 September 2021.